Unraveling the Mechanisms of Homeodynamics and Allostasis, Their Disruption in Living Organisms, and Their Medical Applications
Our primary goal is to deepen the understanding of the dynamic mechanisms underlying the expression of biological functions through interdisciplinary research in the field of biomedical engineering and informatics. We aim to establish a foundation in informatics and systems science that contributes to medical research focused on elucidating the disease mechanisms that alter biological functions due to illness. In particular, we focus on elucidating the biological control mechanisms behind homeodynamics and allostasis, which are modern interpretations of homeostasis—the property of maintaining the "optimal state" of a living organism—using specific biological functions as research subjects. Additionally, we are conducting research to understand the mechanisms of diseases (dynamic diseases) caused by the destabilization of homeodynamics and to develop medical system foundations that enable quantitative diagnostic support for these diseases based on biological control mechanisms.
References
- Tschantz, A., Barca, L., Maisto, D., Buckley, C.L., Seth, A.K. and Pezzulo, G. (2022) Simulating homeostatic, allostatic and goal-directed forms of interoceptive control using active inference, Biological Psychology, Vol. 169, 108266, https://doi.org/10.1016/j.biopsycho.2022.108266.
- Rabinovich, M.I., Simmons, A.N. and Varona, P. (2015) Dynamical bridge between brain and mind. Trends in Cognitive Sciences, Vol. 19 (8), pp. 453-461, https://doi.org/10.1016/j.tics.2015.06.005.
Model-Based and Data-Driven Approaches to Biological and Brain Functions:
To achieve the aforementioned goals, we observe and acquire mesoscopic or macroscopic scale biological time-series signals, specifically body movement and brain activity data, and quantify their complex variations, such as biological fluctuations and rhythms. This data-driven approach in our research promotes the development of machine learning devices and dynamic biomarkers. These innovations enable the classification of healthy individuals and patients based on the characteristics of biological fluctuations and help quantify the severity of patients' diseases.
On the other hand, identifying the dynamic control systems that generate the observed biological fluctuations and mathematically analyzing their nonlinear dynamics through a model-based approach is a core challenge in our research. Dynamic models assimilated with observed data can explain the mechanisms of healthy function expression accompanied by biological fluctuations and predict the onset, progression, and effects of medical interventions on diseases manifested by changes in biological fluctuations. In this context, many dynamic models are identified as reinforcement learning systems or model predictive control systems that achieve the "optimal state" realized by homeodynamics and allostasis. Interestingly, the functional expression strategies and control modes acquired in environments filled with factors that induce instability in biological system dynamics, such as exogenous and endogenous noise and unavoidable delays in biological feedback control, often possess both flexibility and robustness through ingenious mechanisms. Learning new control modes from living organisms, fundamentally different from those used in artificial engineering devices, is also one of our research objectives.
Developing an integrated approach of model-based and data-driven research is one of the most important challenges in 21st-century informatics and systems science. We aim to contribute to solving various problems in the field of biomedical engineering and informatics while envisioning such an integrated approach.
References
- Takazawa, T., Suzuki, Y., Nakamura, A., Matsuo, R., Morasso, P. and Nomura, T. (2024) How the brain can be trained to achieve an intermittent control strategy for stabilizing quiet stance by means of reinforcement learning. Biological Cybernetics, Vol. 118, https://doi.org/10.1007/s00422-024-00993-0
- Bahuguna, J. Verstynen, T. and Rubin J.E. (2024) How cortico-basal ganglia-thalamic subnetworks can shift decision policies to maximize reward rate. bioRxiv 2024.05.21.595174; doi: https://doi.org/10.1101/2024.05.21.595174
Elucidating the Brain Mechanisms of Motor Dysfunction in Parkinson's Disease:
One of our primary research subjects is motor dysfunction caused by Parkinson's disease. It has been recognized that the destabilization of limb, trunk, and eye postures, including standing posture, as well as the destabilization of gait caused by Parkinson's disease, can be regarded as dynamic diseases arising from the destabilization of homeodynamics. Parkinson's disease is a disorder of the basal ganglia, the seat of reinforcement learning, where information processing plays a crucial role in maintaining body posture and realizing and impairing gait functions. We aim to elucidate the brain mechanisms of motor dysfunction in Parkinson's disease patients by identifying brain control systems for body movements based on motion measurement, electroencephalography (EEG), and electromyography (EMG) measurements, and their time-series data. Furthermore, we employ molecular imaging techniques using magnetic resonance imaging (MRI) to visualize the spatiotemporal dynamics of molecules within the body. This involves developing highly sensitive measurement methods and rapid imaging techniques for the brain distribution of dopamine, known to decrease in the brains of Parkinson's disease patients.
References
- Suzuki, Y., Nakamura, A., Milosevic, M., Nomura, K., Tanahashi, T., Endo, T., Sakoda, S., Morasso, P. and Nomura, T. (2020) Postural instability via a loss of intermittent control in elderly and patients with Parkinson’s disease: A model-based and data-driven approach. Chaos, Vol. 30 (11), art. 113140. https://doi.org/10.1063/5.0022319
- Xiang, M., Glasauer, S. and Seemungal, B.M. (2018) Quantitative postural models as biomarkers of balance in Parkinson's disease. Brain, Vol. 141(10), pp. 2824-2827. https://doi.org/10.1093/brain/awy250
- Gut, N.K. and Winn, P. (2016), The pedunculopontine tegmental nucleus—A functional hypothesis from the comparative literature. Movement Disorders, Vol. 31, pp. 615-624. https://doi.org/10.1002/mds.26556
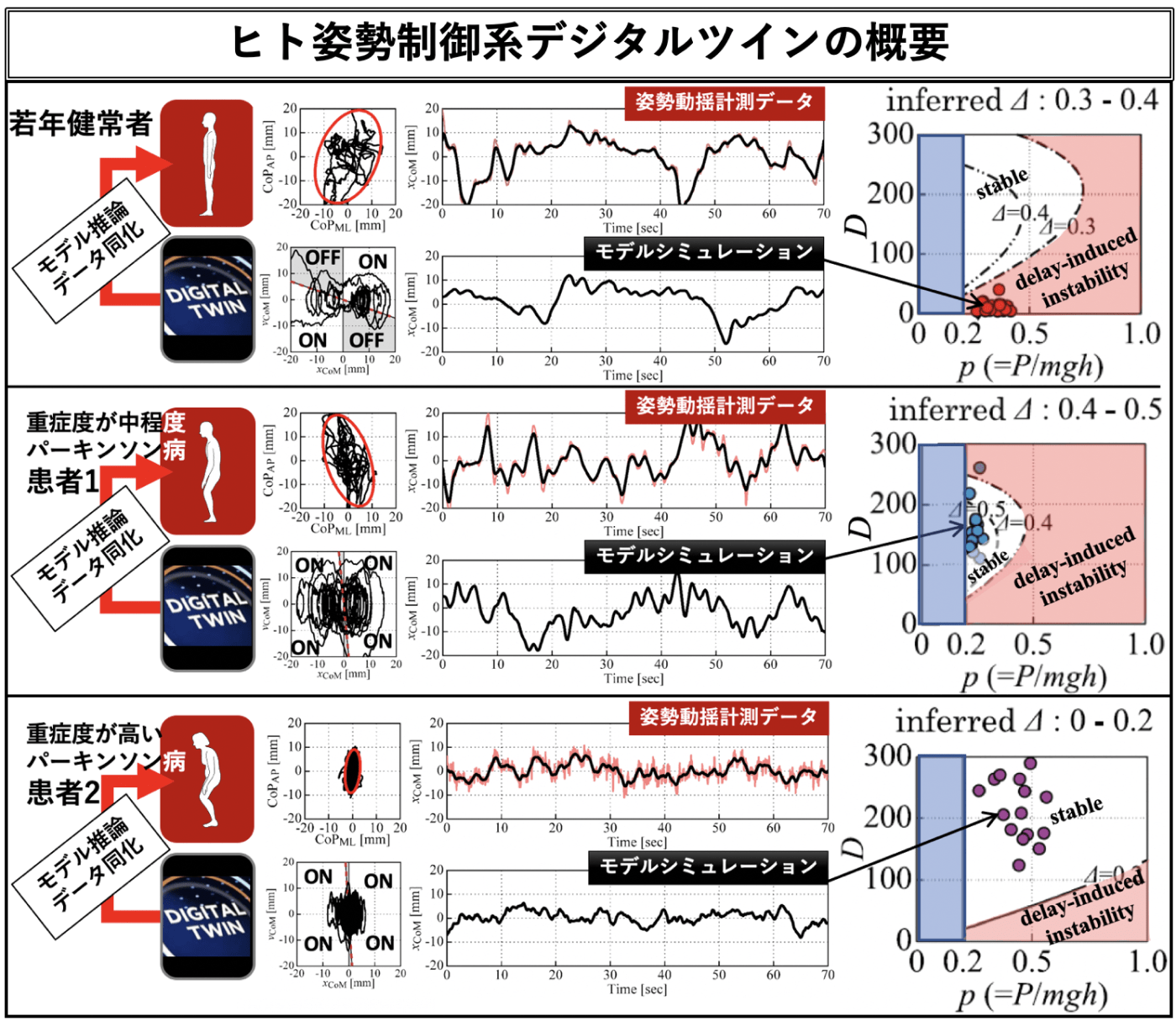
FIG : Analysis of postural fluctuations based on a Digital Twin developed for human postural control, and elucidation of mechanisms of postural function alterations due to Parkinson's disease (Suzuki et al, Chaos Vol. 30, 113140, 2020)